How Explainable AI Can Boost Customer Retention
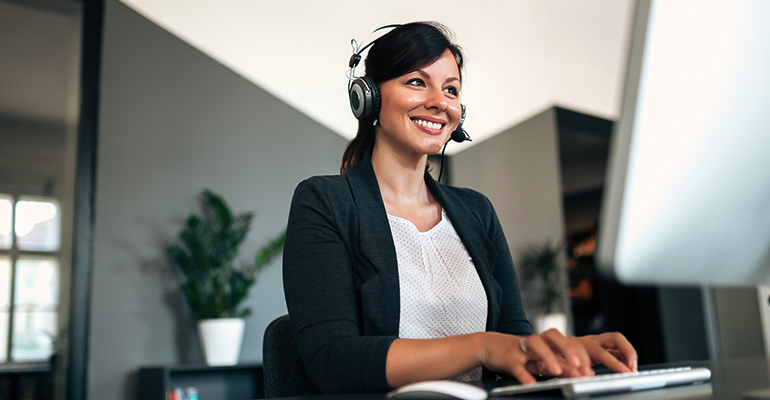
How Explainable AI Can Boost Customer Retention
Fighting churn isn’t about predicting it alone. It also involves understanding the hidden factors that lead to churn and how to drive customer loyalty. Although traditional churn prediction models may be accurate, they often leave businesses in the dark about the reasoning behind their predictions. That’s why there’s a need for explainable AI, as it helps make models transparent and understandable and empowers businesses with actionable insights.
How Explainable AI Helps Drive Retention
- It instills confidence by providing transparency regarding the decision-making process
- It validates the relevance of the model by ensuring it aligns with business objectives.
- It helps identify and rectify any improper variables that might influence the model.
- It provides a deeper understanding of the contextual insights that affect customer behavior and retention dynamics.
Let’s dive deeper into the positive impact of explainable AI on customer retention strategies.
Identifying Risk Indicators
A customer often defects after a series of negative experiences, but they exhibit risk signals long before they decide to leave. Using explainable AI, you can continuously monitor their journey and identify specific risk indicators that contribute significantly to churn. These indicators may include a decline in product usage, a history of unresolved issues or frequent mentions of competitors. This allows you to minimize churn risk with proactive intervention, instead of reacting to it when the customer expresses it.
Making Customer-Level Predictions
Explainable AI helps you with churn predictions down to the individual customer level. Using risk indicators, it can provide detailed insights into which customer is likely to churn and why. Let’s say you’re a home security business. Your explainable AI platform indicates that a particular customer is 80% likely to churn. It also tells you that they haven’t used your automation system in the last few weeks and they have a history of issues with their security camera. Now, you know what’s primarily driving the risk and can tailor a retention strategy for that customer.
Providing Increased Transparency
Unlike the black box approach, in which you don’t have visibility into how a model arrived at its prediction, explainable AI provides clarity and interpretability of its predictions, explaining the factors that drive churn likelihood and the rationale behind its recommendations. This transparency enables effective decision-making built on trust and confidence.
For example, besides predicting the churn risk level for a specific customer segment, it can explain that the prediction is based on frequent calls from the segment regarding billing issues and non-renewal of the subscription plan. It can further recommend that you offer those customers a discounted, customized subscription plan.
Enabling Actionable Decisions
Explainable AI’s transparency not only allows for uncovering the rationale behind churn predictions but also leverages that rationale to make actionable decisions that don’t involve any guesswork. Backed by understandable reasons, the decisions resonate with customers and drive measurable results on revenue, growth, customer lifetime value and brand reputation. This enables you to detect and address issues proactively and identify opportunities to improve products, optimize campaigns, allocate resources effectively and build a customer-centric culture.
Analyzing Sentiments
Not all customers express their dissatisfaction. Neither do all negative sentiments pose equal churn risk. That’s why sentiment analysis is critical for effective retention. An explainable model can also provide deeper insights into the factors influencing customer sentiments, such as customer service, on-call experience, product quality and pricing. For example, you can use explainable AI to analyze customer feedback, agent notes, service history and product usage to detect patterns of customer satisfaction or dissatisfaction. You can then take proactive measures to address the root causes of negative sentiments and improve overall customer experience, thereby boosting retention.
Recommending Targeted Offers
Risk drivers and levels are different for all at-risk customers—and so the retention offers should be too. A customer leaving due to poor service history won’t be interested in a discount on the subscription plan. Using the risk profile of at-risk customers, explainable AI can help you create personalized offers that align with their needs and preferences. Targeted offers stand a higher chance of acceptance, which results in an improved retention rate. Furthermore, more personalized and engaging interactions with customers, driven by explainable AI, demonstrate that you understand and value them, and this can lead to improved satisfaction and loyalty.
Empowering Agents
Explainable AI can dramatically change the role of your contact center agents from passive employees to customer retention champions by giving them all the relevant customer insights they need right on their screen. Armed with the risk profile, contextual information and recommended offers for every customer that calls, your agents can engage with customers in genuine conversations that build rapport and trust, increasing the likelihood of retention. Also, coupling this with giving them decision-making authority can significantly reduce wait times.
How To Get Started
Implementing explainable AI in your retention strategy doesn’t require a complete data science overhaul. Here are the steps to follow:
- Unify customer data from all sources, such as the CRM, contact center, sales, service history, and product usage databases. Apply machine learning models to this multi-structured customer data to generate powerful AI insights.
- Uncover hidden patterns to diagnose the factors driving customer dissatisfaction and forecast future behavior based on the identified risk drivers.
- Segment customers based on common risk drivers to design targeted offers and interventions for each risk segment.
- Empower your frontline, including your contact center and field service agents, to take action using intelligence that includes risk scores, contextual information, and suggested offers.
Explainable AI isn’t a one-time project but a continuous journey. Once set in motion, explainable AI continues to improve model performance. It helps you analyze the impact of decisions, refine the models, gain a deeper understanding of your customers, and fine-tune your interventions. This can lead to more accurate and effective models delivering continuously better retention results.