The power of AI for personalizing CX
How to use AI for customer behavior analysis for deeper insights that can offer enhanced content, product recommendations and pricing - ultimately refining customer experiences across sectors
Add bookmark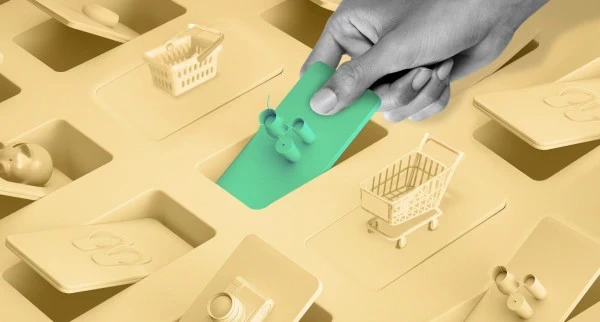
Too many customer-personalization efforts are stuck in what you might call the census bureau approach.
A company knows your gender, age, occupation, and birthday. So they send out so-called personalized email blasts—to you and thousands of other people just like you in your market segmentation—hoping that you’ll bite at a sales promotion they created just for you.
Fat chance, says Dan Gingiss, former digital marketing chief at Humana and now a customer experience consultant. “Just knowing a bunch of variables and data points about somebody isn't personalization,” says Gingiss, whose clients include IKEA, Samsung, and Adobe. “Real personalization is knowing I am a big baseball fan and have rooted for the Cubs my whole life, that I play pinball and pickleball, have two kids in high school, and travel a lot for work.”
AI is bringing companies closer to that ideal—and closer to their customers. AI techniques like machine learning can analyze customer behavior, sentiment, demographics, and purchase histories. These benefits are delivering results as companies learn how to use the tools. Strategic advisory firm NewVantage Partners reported last year that 92 percent of companies investing in AI technology had experienced measurable results, up from just 48 percent five years prior.
Here are some of the most common use cases for personalization strategies and how AI is impacting each.
Content personalization
Customized messaging and promotions for specific customer segments
Conventional strategy
Traditional marketing personalization relies on persona-based segmentation that divides users into a series of broad demographics (e.g., age, income, occupation). But, as Gingiss points out, that data isn’t truly personal. It doesn’t appeal to an individual’s passions and lifestyle.
The AI advantage
Greater insight comes from data, but it's more detailed and delivered in greater volume and at higher velocity.
AI algorithms train on vast amounts of data such as customer demographics, page views, and downloads. The models spot patterns such as the fact that men in a certain age range who play sports really like to view a particular subset of product videos, but only on mobile. They can use that to tailor their content strategies.
The streaming giant Spotify has long used machine learning to understand its listeners, explains the company on its R&D site. Senior leader of personalization Oskar Stål detailed how analyzing their streaming histories (e.g., the artists, genres, and even the number of beats per minute in the songs they like) enables the company to deliver a weekly digest of personalized music content. Spotify has also explained how it is adding a generative AI “DJ” that will play custom content for listeners with no prompting, along with commentary on the artists and songs, in a realistic automated voice.
Key sector
Wherever content is the primary product (think: media and entertainment). For example, Timothy Whitney, senior owner of product data analytics, and Stephen Shepherd, staff data engineer at Warner Bros Discovery, explained how the company has invested heavily in AI-driven content recommendation systems to help viewers discover relevant new content based on their viewing habits. In the first four months of implementation, users exposed to the personalization system averaged up to 14 percent higher engagement with recommended shows than randomized control users.
Product recommendations
When a customer is shown specific products in which they may be interested, based on their past browsing and purchase history
Conventional strategy
Companies use consumers’ online search histories to understand the categories of products that interest buyers, potential buyers, and buyers with similar search histories.
The process, known as collaborative filtering, often fails to recommend new products because the system doesn’t know enough about the product to recommend it. A specialist e-commerce site can’t recommend any handbag just because the customer is looking at handbags; products need to match precise criteria such as color, shape, style, and intended audience segment.
The AI advantage
AI thrives on data, but that doesn't just mean customer data. Companies must build more accurate models of their product portfolios to feed their AI personalization engines, says knowledge strategist Seth Earley, CEO of Earley Information Science and author of The AI-Powered Enterprise. “The product information models need to align with what's important to the user,” he says.
That accuracy can come from more detailed data on the products themselves. That could include the full text from a user manual or the operating parameters of a refrigerator, along with its unique lifestyle features and energy rating.
This content could appeal to different types of customers in different circumstances. An affluent customer who loves tech might care more about a fridge’s built-in screen and internet connectivity than about annual electrical usage. An amateur mixologist might want to know about its ice maker.
Key sector
Product recommendations are key in e-commerce, where companies must help consumers find the right product fast and with the least amount of friction.
Fashion-focused e-commerce retailer ASOS, which sells more than 850 brands online, uses collaborative filtering but goes beyond that. On its tech blog, machine learning scientist Jacob Lang explains that when the company adds a new product to its site, it first uses neural networks to analyze pictures and descriptive text of the product, identifying attributes such as style, color, price range, pattern, market segment, and seasonality.
The AI then maps these characteristics against product features that are most likely to appeal to each customer, bringing new products into each customer's recommendations field immediately.
ASOS also provides a feature in its mobile app called Style Match. Shoppers upload images of clothing they like, then Style Match uses AI to “look” at the images and find product matches with similar colors and styles.
Dynamic pricing
When prices are adjusted according to the customer's purchasing habits or competitors’ prices
Conventional strategy
Companies sometimes use their own inventory data or competitor pricing data to determine what to charge for a product or service. Rules-based pricing systems use relatively slow-moving information such as inventory levels and rely heavily on historical data such as sales figures, extending back for multiple business cycles, for their calculations.
RELATED CONTENT: A glimpse at the future of AI-powered sales
These are often reviewed by a human industry expert who uses their own industry experience to sanity-check the results. The result is reactive pricing that often doesn't anticipate future events such as the effect of supply chain disruptions and competitors’ price changes on demand.
The AI advantage
AI-powered pricing systems take more types of data on board in real time. This data depends on the industry but can include anything from the weather and the customer's location to road traffic congestion. The AI uses this data to adjust a product or service price down to a regional or even an individual customer level, and within minutes.
Key sector
The more volatile prices are in an industry, the more relevant AI-powered personalization can be. Uber uses machine learning to adjust fares on the fly, factoring in time of day, your personal ride history, and even your phone's battery life: If you're running low on battery, you're more likely to accept a higher “surge fare” than to wait and try to land a lower fare.
Behavioral personalization
When a customer's behavior influences their interaction with the company
Conventional strategy
Purchasing history has long been the most reliable data set for determining consumer behavior. But that data misses a lot of crucial information about the customer’s journey on their way to clicking the “buy now” button.
The AI advantage
AI allows companies to gather and crunch more data about consumers—who are spending more time online and leaving behind more personal digital breadcrumbs—in real time.
These captured behaviors range from consumers’ website navigation habits to the amount of time spent on a particular webpage. They also capture user interactions with specific features in a brand's mobile app, when that app was accessed, and from where.
Companies are marrying this digital telemetry with sentiment analysis gleaned from a customer's messages or from their tone of voice on a phone call. This enables a new level of predictive analytics, says marketing technology and digital transformation consultant Greg Kihlström.
“It feeds the concept of 'next best action,' where you build propensity models based on what the customer has done and then using it to decide what we show them next," he says.
Key sector
Any industry that interacts with online users, from cellular carriers looking to provide best-in-class customer support to financial service providers that are trying to understand customers' pending life decisions and their financial needs.
We want to hear from you! Please send us your feedback, and get informed about exciting updates from The Works. Drop us a line: theworks@freshworks.com.